Unlocking the Potential of Image Classification Annotation Tools
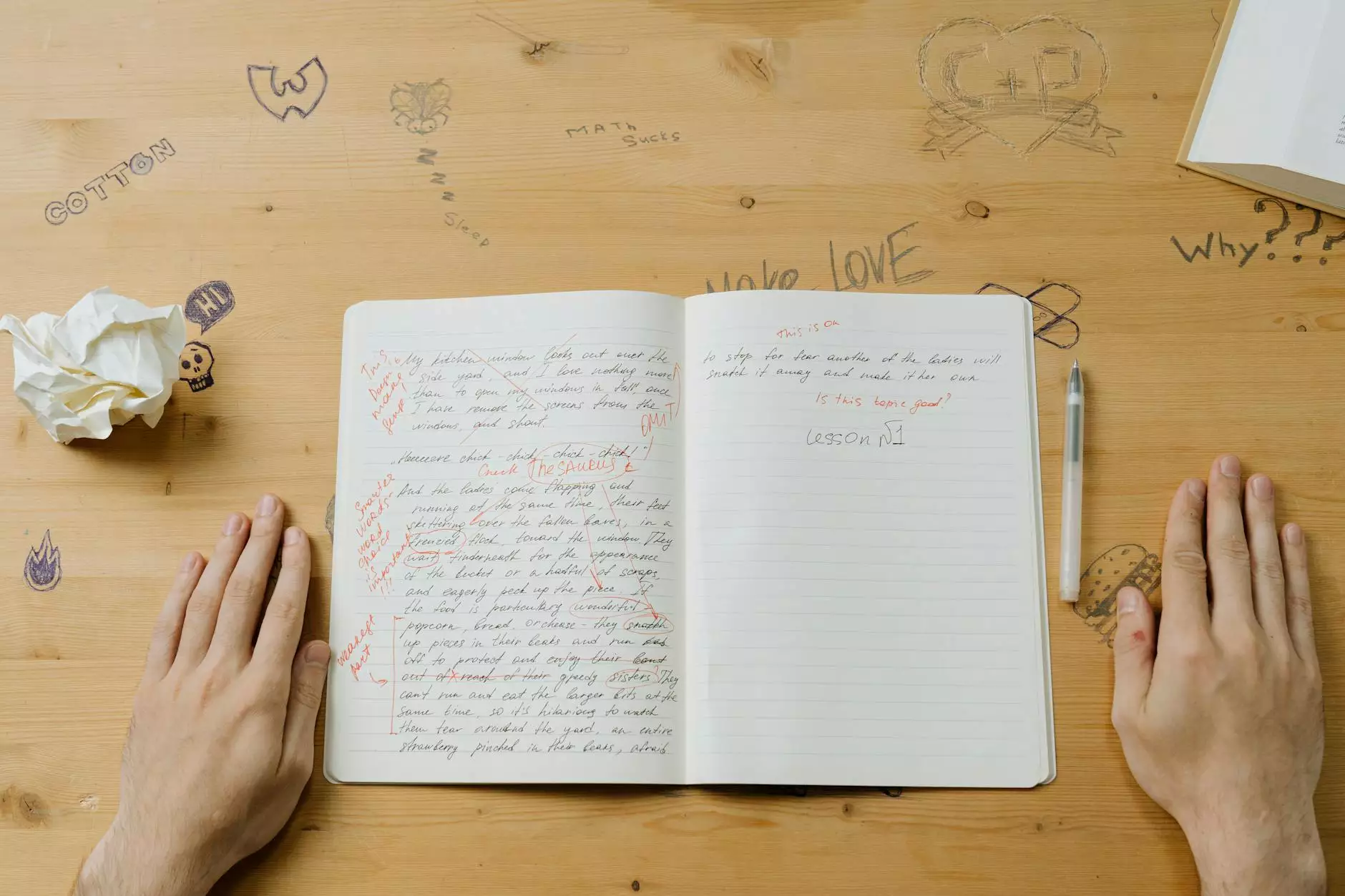
In the world of artificial intelligence and machine learning, the relevance of data cannot be overstated. As businesses strive to harness the power of AI, the need for precise and efficient data annotation becomes paramount. One crucial aspect of this process is the use of image classification annotation tools, which streamline the categorization of visual data, making it easier for algorithms to learn and evolve.
Understanding Image Classification Annotation Tools
Image classification annotation tools are designed to facilitate the tagging of images with predefined categories. These tools allow data scientists and machine learning practitioners to manually or semi-automatically label images, providing the necessary input for training powerful machine learning models. The effectiveness of these tools has a direct impact on the quality of the output generated by AI systems.
What is Image Classification?
At its core, image classification is the process of identifying and categorizing objects within an image. This is achieved by assigning labels based on specific features found in the visuals. For instance, an image classification algorithm might differentiate between pictures of cats, dogs, and other animals.
The Role of Annotation in Machine Learning
In the realm of machine learning, annotation refers to the process of labeling data, which in this case, involves marking images with relevant tags or categories. This labeled data serves as a training set, enabling machine learning models to recognize patterns and improve their accuracy over time.
Key Features of an Effective Image Classification Annotation Tool
An effective image classification annotation tool should possess several essential features:
- User-Friendly Interface: A simple and intuitive design allows users to annotate images quickly and efficiently without a steep learning curve.
- Robust Annotation Options: Tools should support various annotation types, including bounding boxes, polygons, and semantic segmentation.
- Collaboration Features: The ability to work in teams and share projects easily can significantly enhance productivity.
- Integration Capabilities: Compatibility with other software platforms and machine learning frameworks is crucial for seamless workflows.
- Quality Assurance Mechanisms: Automated checks and balances can help maintain the accuracy of annotations.
Benefits of Using Image Classification Annotation Tools
Implementing image classification annotation tools can bring numerous advantages to businesses involved in AI development. Here are a few of the standout benefits:
Improved Data Quality
One of the primary benefits is the enhancement of data quality. High-quality annotations lead to more accurate machine learning models, reducing the likelihood of errors and improving overall performance.
Efficiency in Data Processing
Using an effective tool can drastically speed up the data annotation process. Automation features within these tools mean that less time is spent on manual labeling, allowing teams to focus on more complex tasks.
Scalability
As businesses grow and their data needs increase, having a reliable annotation tool ensures that scaling up processes can be achieved without compromising quality or speed.
How to Choose the Right Image Classification Annotation Tool
With numerous options available in the market, selecting the right image classification annotation tool can be challenging. Here are key factors to consider:
Assess Your Needs
Clearly outline the specific requirements of your project. Consider the types of images you are working with and the level of detail needed in annotations.
Evaluate Tool Features
Look for tools that offer a rich set of features tailored to your needs. Focus on those that simplify the annotation process, facilitate collaboration, and include robust quality control.
Check for Integration
Ensure that the tool can integrate seamlessly with your existing systems and workflows. This will save time and reduce disruptions as you transition to new software.
Consider User Feedback and Reviews
Research user reviews and testimonials to gauge the tool’s performance and reliability. Feedback from other users provides valuable insights into the tool’s effectiveness and customer support.
Popular Image Classification Annotation Tools Available Today
Here are some well-regarded tools for image classification:
- Labelbox: A collaborative platform that offers a variety of annotation options and integrates easily with different machine learning frameworks.
- VGG Image Annotator (VIA): An open-source tool that is lightweight and user-friendly, ideal for users who seek simplicity.
- RectLabel: Great for macOS users, this tool supports several annotation types and is praised for its ease of use.
- SuperAnnotate: This comprehensive tool excels in collaboration and boasts advanced automation features for efficient workflows.
Best Practices for Image Annotation
To get the most out of your image classification annotation tool, consider following these best practices:
Define Clear Labeling Guidelines
Creating a set of guidelines for how to label images ensures consistency across your dataset. This includes defining category names, determining edge cases, and setting expectations for annotation quality.
Conduct Regular Training Sessions
Educate your annotators about the intricacies of the images and the topics at hand. Regular training helps enhance their skills and improves overall annotation quality.
Utilize Quality Control Measures
Introducing quality control checkpoints within your annotation process helps identify inconsistencies and maintain high standards throughout the project.
The Future of Image Classification Annotation Tools
As technology advances, the landscape of image classification annotation tools continues to evolve. Emerging trends include:
Increased Automation
Future tools are likely to integrate greater levels of automation, reducing the need for human input and speeding up the overall annotation process.
Machine Learning Enhancements
Tools that use AI to assist in the annotation process, suggesting labels based on previous input, will significantly improve efficiency and accuracy.
Collaborative Platforms
As teams become more remote, tools designed for collaboration will continue to gain traction, allowing for real-time updates and team communication.
Conclusion
In conclusion, image classification annotation tools are vital assets in the data annotation landscape. By investing in the right tools and adhering to best practices, businesses can significantly enhance their machine learning projects. As the demand for high-quality data continues to rise, the importance of mastering these tools cannot be understated.
Explore more about effective annotation solutions at keylabs.ai and empower your data-driven journey today!